Autograd¶
Autograd는 자동 미분을 수행하는 torch의 핵심 패키지로, 자동 미분을 위해 테잎(tape) 기반 시스템을 사용합니다.
순전파(forward) 단계에서 autograd 테잎은 수행하는 모든 연산을 기억합니다. 그리고, 역전파(backward) 단계에서 연산들을 재생(replay)합니다.
변수(Variable)¶
Autograd에서 우리는 Variable
클래스를 만나볼 수 있는데요, 이는
Tensor
를 매우 얇게 감싸고(wrapper) 있습니다.
.data
속성을 사용하여 tensor 자체(raw tensor)에 접근할 수 있으며,
역전파 연산이 끝나고 나면, 이 변수에 대한 변화도(gradient)는 .grad
에
누적됩니다.
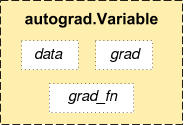
Variable
Autograd 구현에서 매우 중요한 클래스가 하나 더 있는데요, 바로 Function
클래스입니다.
Variable
과 Function
은 상호 연결되어 있으며,
모든 연산 과정을 부호화(encode)하여 순환하지 않은 그래프(acyclic graph)를 생성합니다.
각 변수는 .grad_fn
속성을 갖고 있는데, 이는 Variable
을 생성한 Function
을
참조하고 있습니다. (단, 사용자가 만든 Variable은 예외로, 이 때 grad_fn
은
None
입니다.)
도함수를 계산하기 위해서는, Variable
의 .backward()
를 호출하면 됩니다.
Variable
이 스칼라(scalar)인 경우(예. 하나의 요소만 갖는 등)에는, backward
에
인자를 정해줄 필요가 없습니다. 하지만 여러 개의 요소를 갖고 있을
때는 tensor의 모양을 gradient
의 인자로 지정할 필요가 있습니다.
import torch
from torch.autograd import Variable
x = Variable(torch.ones(2, 2), requires_grad=True)
print(x) # notice the "Variable containing" line
Out:
Variable containing:
1 1
1 1
[torch.FloatTensor of size 2x2]
print(x.data)
Out:
1 1
1 1
[torch.FloatTensor of size 2x2]
print(x.grad)
Out:
None
print(x.grad_fn) # we've created x ourselves
Out:
None
변수 x에 연산을 수행합니다:
y = x + 2
print(y)
Out:
Variable containing:
3 3
3 3
[torch.FloatTensor of size 2x2]
y 는 연산의 결과로 생성된 것이므로, grad_fn 을 갖습니다.
print(y.grad_fn)
Out:
<AddBackward0 object at 0x7fc227d9f550>
y에 다른 연산을 수행합니다:
z = y * y * 3
out = z.mean()
print(z, out)
Out:
Variable containing:
27 27
27 27
[torch.FloatTensor of size 2x2]
Variable containing:
27
[torch.FloatTensor of size 1]
변화도(Gradient)¶
이제 역전파(backprop)를 하고 변화도 d(out)/dx를 출력해보겠습니다.
out.backward()
print(x.grad)
Out:
Variable containing:
4.5000 4.5000
4.5000 4.5000
[torch.FloatTensor of size 2x2]
기본적으로 변화도 연산은 그래프 상의 모든 내부 버퍼를 새로 쓰기(flush) 때문에,
그래프의 특정 부분에 대해서 역전파 연산을 2번하고 싶다면, 첫 연산 단계에서
retain_variables = True
값을 넘겨줘야 합니다.
x = Variable(torch.ones(2, 2), requires_grad=True)
y = x + 2
y.backward(torch.ones(2, 2), retain_graph=True)
# retain_variables 플래그(flag)는 내부 버퍼가 사라지는 것을 막아줍니다.
print(x.grad)
Out:
Variable containing:
1 1
1 1
[torch.FloatTensor of size 2x2]
z = y * y
print(z)
Out:
Variable containing:
9 9
9 9
[torch.FloatTensor of size 2x2]
무작위 값으로 역전파를 합니다.
gradient = torch.randn(2, 2)
# retain_variable 을 지정하지 않았다면 오류가 발생할 것입니다.
y.backward(gradient)
print(x.grad)
Out:
Variable containing:
0.1651 0.9929
-0.6825 2.6321
[torch.FloatTensor of size 2x2]
Total running time of the script: ( 0 minutes 0.002 seconds)